OCR Training Dataset For Deep Learning Models
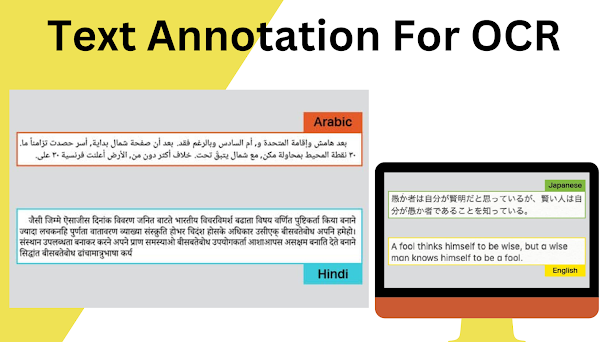
Introduction
Before the 2012 revolution in deep learning, various OCR implementations were available. OCR is still a complex issue, even though it was generally believed to have been solved. It is mainly the case when text images are taken in an uncontrolled environment. I'm referring to the images' complex backgrounds, including lightning, noise, various fonts, and geometrical aberrations. However, numerous datasets are available in English, and finding data for different languages can take time and effort. Different datasets present different issues that need to be resolved. Here are some examples of the kinds of data that are commonly used to address machine learning OCR problems.
This Information Age is all about AI. It's great for people who use it and also helps businesses grow, and it's generally one the clear evidence of the progress of the human race, where machines perform many mundane or tedious tasks. Technology is growing with newer and more efficient ways to improve the efficiency of our world. However, deep learning is less popular with the general public compared with artificial intelligence and its intelligent child machine learning.
1. SVHN The Data Set
The Street View, House Numbers dataset includes 26032 digits to test as well as 73257 digits used for training and 531131 digits to train. Ten labels, numbered from 0 through 9, have been contained in the data set. The data set is distinct from MNIST because SVHN has pictures of house numbers framed against different backgrounds. Contrary to MNIST's numerous images of numbers, This Dataset For Machine Learning includes bounding boxes for each number.

2. Scene Text Data Set:
The dataset consists of 3000 images with texts written in Korean and English displayed in different outdoor and indoor settings as well as lighting conditions. In addition, specific photos are accompanied by numbers.
3. Devanagari The Character Data set
With the assistance of 25 writers from the local community using the Devanagari script, This collection gives us 1800 examples of 36-character classes. Many more are available, such as those that support Chinese characters, CAPTCHA, and handwritten texts.
OCR Learning Dataset
It is much easier to save documents, update, index and search for information in an electronic document in this digital age than to spend long hours scrolling through printed, handwritten or written documents. Furthermore, scrolling manually through the pages of a massive non-digital document to search for information takes time. It increases the possibility that we will need help to locate the details. For our benefit, technology is becoming better and better at completing things that people used to believe they could not complete by themselves. They often outperform us too.
Text detection algorithms are required to locate the text within an image and draw a bounding line around the text's area. It is also capable of detecting this type of objection with conventional techniques.
1. Sliding Window Technique:
The sliding window method can create the bounding box surrounding the text. It involves a significant amount of computing. Like Convolutional neural networks, this method uses a window sliding to move through an image and identify the text within the frame. We make sure to use a variety of sizes of windows to ensure that the text area is noticed. The sliding window is an implementation of convolution that could accelerate calculations.

2. Single shot and detectors based on region
In contrast to the sliding window, the YOLO strategy is based on one sweep across the image to find the text. This strategy based on region is comprised of two steps. The network suggests a location that could include the test and then decides if that area contains the test. To better understand the methods used for object detection, which is, in this instance, the detection of text, visit one of my earlier posts. Artificial data synthesizing makes new data objects accurately matched to accurate data. Actual data is gathered from surveys or directly measured in the real world. Fake data are those that are obtained as a result of computer-generated simulations. The most important thing to consider is the statistics of the data source being reproduced in the created data.
Artificial Data Synthesis has several various reasons to use it. In the introduction, the primary goal is to boost algorithms' efficiency by increasing the training's size. However, It can protect sensitive information such as Personal Identifiable Information (PII) or Personal Health Information (PHI). In any situation when data is difficult or costly to collect, artificial data can be the main factor in determining the value. Identifying anomalies or fraud is a good illustration of this: although the evidence of fraudulent transactions is uncommon, it could be possible to make fraudulent transactions. Artificial data can be particularly beneficial in Optical Character Recognition (OCR), in which the amount of OCR Training Dataset used in the model is essential to a model's overall accuracy.
I split these data sets into test and train sets before deriving the role to enhance the data. The image, and the vector that defines the direction of the shift and its magnitude, are used as inputs. The shift causes some of the pixels to become empty. I recommend studying this guide to get started since this guide explains Random Forest classifier's operating principles are different from the subject of this post. A Random Forest is, in essence, a set of Decision Trees. The trees are trained using various random parts of the training set to guarantee diversity (with and without replacement). Random Forest makes an aggregate forecast following the majority of votes and accounts for the weak classifier's decisions.
The control of artificial intelligence is too far away. OCR is among its most significant points. Everyone is conscious that optical recognition is a; however, until recently, we could not see the work behind the scenes and the unique optical character recognition technique. It's easy to find the character, eliminate it, or even optimize documents so that they are readable by machines. We've scanned some of our documents to satisfy an official reason. It is only possible by OCR. The scans are essential to building OCR data sets, which can then extract data from handwritten documents like invoices, receipts, bills, and many other documents. We have discovered that, without a well-structured dataset, any OCR system will be effective and efficient; we're ready to offer you specialized client training.

Outsource your OCR TRAINING DATASET from GTS.AI
Global Technology Solutions (GTS.AI) has got your business covered with premium quality dataset. With its remarkable accuracy of more than 90% and fast real-time results, GTS helps businesses automate their data extraction processes. In mere seconds, the banking industry, e-commerce, digital payment services, document verification, barcode scanning, Image Data Collection, AI Training Dataset, along with Video Annotation and many more can pull out the user information from any type of document by taking advantage of OCR technology. This reduces the overhead of manual data entry and time taking tasks of data collection.
Comments
Post a Comment