SOME LATEST TECHNIQUES FOR DATA ANNOTATION SERVICES
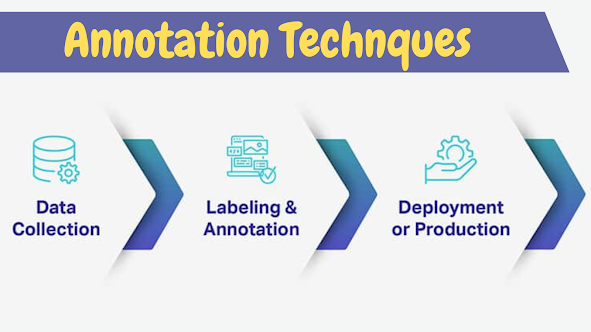
Introduction
GTS.AI will present the latest techniques for the data set. Data annotation is adding labels, categories, and other contextual elements to a data set so that computers can understand and use the data. The GTS can help you understand the procedure. While data annotation is utilized with audiovisual and image elements, the technique is often used using numerical data or alphabetical words in AI and machine learning.
The usage of Data Annotation in applications, and some benefits, that are currently available and likely to come from this method, are described in greater detail below.
GTS on Features of Data Annotation Services
Some of the essential tools enable you to mark up any data:
Ontology: These are the specifications that provide valuable and precise annotation frameworks. Data Annotation Services types, labelling guidelines, and guidelines for class and attribute are just a few examples of the information found in ontology's. Because there are many kinds of raw data available and data sets, selecting "smart" raw information or data relevant to the development of your specific AI tools is essential. In most cases, the data comes from records of historical human interactions, which the organization can access; however, sometimes, open-source data is sufficient. Examples that show bright sets of data: with appropriate examples of data samples, it's possible to perform Annotation of data.
Tools to manage and store data sets Annotation of data for AI and projects that rely on machine learning will require a significant amount of basic information. It is essential to manage and store this data in a file system or a program capable of handling the bandwidth to keep raw and annotated data well-organized and easily accessible.

GTS: Techniques for Data Annotation Services
When making annotations on data, it's crucial to consider the guidelines for Annotation, the size of the set, and the cost per Annotation. Different techniques for Annotation are appropriate depending on the goals of your project and financial circumstances and whether the raw data set is readily available. To help you select the most effective method to implement the best approach to your AI initiative, we've provided some of the AI techniques with their advantages and disadvantages below and provided a brief overview of each.
Handwritten Data Annotation
It is possible to manually annotate any data set in the beginning phases of any AI project, for instance, when the data set isn't huge or when the goal is to build the prototype rapidly. The developers working on the project will look over the data and then add labels to the data samples that conform to the annotation guidelines.
Advantages
- A minimum amount of effort is required to handle the Data Annotation
- Engineers are more knowledgeable about the information than other professionals, which improves the quality.
- Engineers may discover exciting data points that could later use used in an algorithm.
Participatory Data annotation
A cost-effective and efficient method for the data annotation and various image and Video Dataset is crowd sourcing. Various platforms are available for crowd sourcing, including GTS.AI.

Benefits
- Cost-effective
- capable of scaling up to an enormous collection
- Fast
Data Annotation uses
In the ideal scenario, data samples are connected to labels. In this scenario, data annotation isn't required. If there's a straightforward business procedure that generates data, this could occur. Loan applications are submitted by people, for instance, which Risk department specialists review. The bank creates an extensive database of loan applications over time, and notifications of rejection or approval. Decisions on loan allocation in binary form (also known as notifications) are equivalent data labels. Data samples are loans that contain details about income, gender, age, education, gender, and other variables. A scoring model that is used for machine learning can be created by using this information.
Most of the content generated by users is, however, noisy and requires further data cleaning. In this case, annotations of users that are not processed can be used as inputs to a more complicated annotation process, such as manual Annotation or crowd sourcing, which could reduce the cost. It is because of the possibility of partial labels. It means that label synthesis is not required, and only verification and cleaning are needed.
Advantages
- social media tagging
- Affordable or free
- If the business process that generates the data is designed correctly, requires only a little administration.
- capable of scaling up to an enormous collection
AI-Driven Data annotation via API
There are instances when an existing API has the same functionality you would like to integrate into your application. In this case, you can utilize the API to send various samples of data, make predictions, and then apply these predictions as tags for data. Send API-annotated data samples for processing and moderation if the accuracy of the labels used for your application is critical. Initial annotation quality could be better, but this technique dramatically reduces annotation effort. It's like the technique of data annotation based on usage. The cost that comes with this API is the main difference. One can, for instance, utilize an API for sentiment analysis to analyze and score the quality of many texts before implementing a customized method to analyze emotions.

Advantages
- Cheap
- A minimal amount of administration is required.
- capable of scaling up to an enormous collection
Comments
Post a Comment